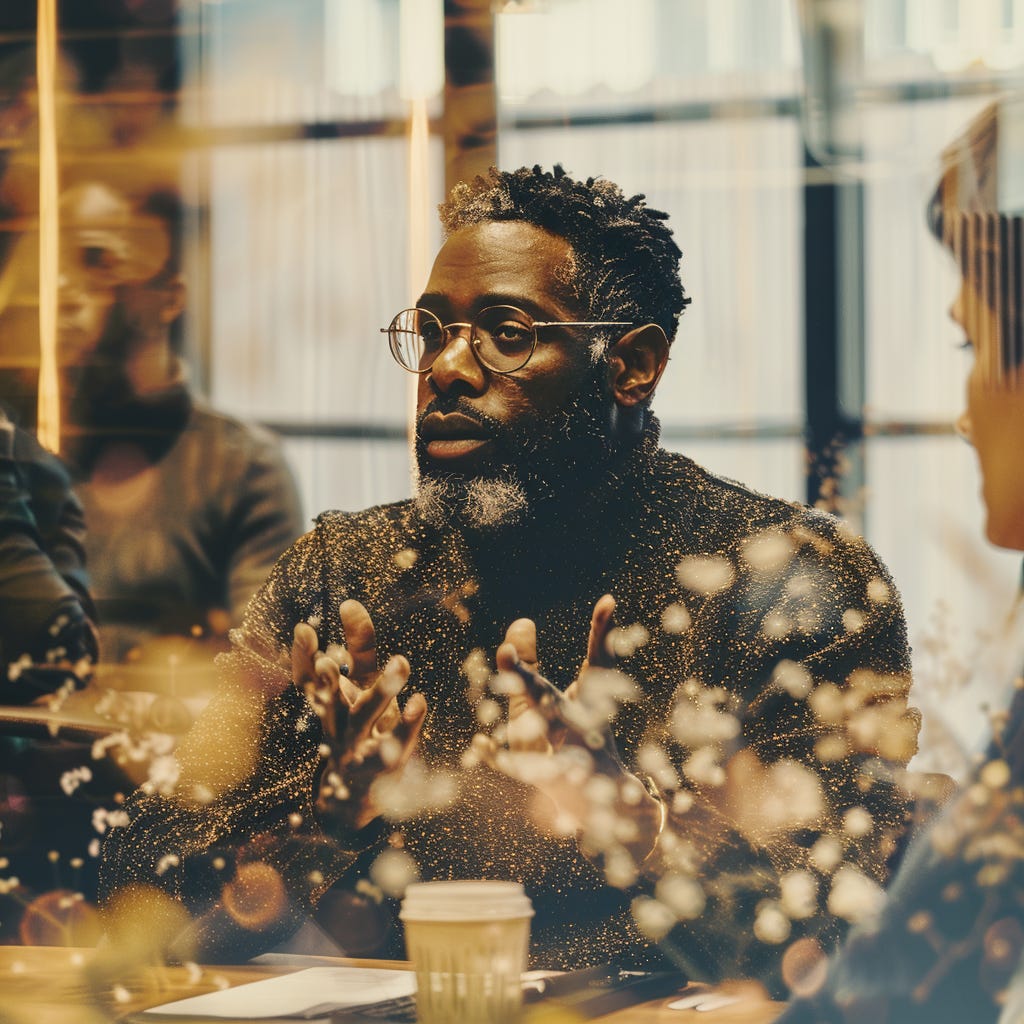
April 10, 2025
The rise of large language models (LLMs) and AI-driven automation marks the death of cost-based competition and the emergence of zero-cost innovation. As AI-native businesses automate execution at near-zero marginal cost, the basis of competitive advantage shifts from cost efficiency to real-time strategic differentiation. This paper develops a theoretical framework to analyze how AI enables instantaneous business model iteration, frictionless entrepreneurial activity, and continuous firm adaptation.
Key insights include:
The disappearance of cost-based competition, forcing firms to compete on architecting unique value propositions rather than operational efficiency.
The rise of infinitely adaptive business models, where AI enables companies to constantly refine their strategic positioning in real time.
An explosion of AI-generated micro-enterprises, reducing barriers to business creation and democratizing innovation.
The shift from execution to strategy, as human labor moves toward designing AI-powered firms rather than operating them.
However, AI-driven business models present new risks, including data monopolization, AI-driven market instability, and regulatory uncertainty. Future research must address how AI-native firms interact with competitive equilibrium, market governance, and labor dynamics.
Ultimately, this paper argues that AI transforms business models from static entities into living, continuously evolving algorithms, marking the arrival of the infinite business model era.
For centuries, economic theory has been built on scarcity—of labor, knowledge, capital, and resources. Firms optimized for cost reduction, efficiency, and competitive advantage through economies of scale (Coase, 1937; Chandler, 1990).
But AI fundamentally changes this. LLMs create infinite intelligence at near-zero cost, removing:
The need for expensive expert knowledge (AI generates reports, strategies, and entire products instantly).
The need for large teams to execute work (automation replaces manual labor).
The need for capital-intensive operations (AI removes complexity in scaling).
This means business models built on cost advantages collapse. The new competitive advantage is who can create the most valuable, strategically unique business model—because execution is free.
How does infinite intelligence (LLMs) create zero-cost innovation, and how does this reshape business value creation?
What happens to competitive dynamics when anyone can design and launch a high-value business at near-zero cost?
Economic models have long assumed that knowledge production is costly and constrained (Arrow, 1962; Romer, 1990). LLMs completely destroy this assumption:
AI enables costless creation of strategic insights, removing the traditional trade-off between knowledge creation and execution.
This leads to zero-cost innovation cycles—firms iterate business models instantly, leading to rapid and unpredictable market shifts.
McAfee & Brynjolfsson (2017) discuss how AI-driven automation leads to marginal cost reduction approaching zero in digital goods.
Standard microeconomic models assume firms compete on marginal cost (Varian, 2010). AI obliterates this dynamic:
When execution is free, cost-based competition disappears.
Product differentiation and strategic design become the only viable competitive advantage.
This aligns with Blue Ocean Strategy (Kim & Mauborgne, 2005)—but now, anyone can create a Blue Ocean instantly, making differentiation ubiquitous.
The shift is not just about cost efficiency—it’s about who owns intelligence-based strategic positioning:
Doval (2022) describes dynamic matching markets, where businesses continuously reposition themselves in real time.
Wolitzky (2016) explores mechanism design under uncertainty, similar to how AI-native firms constantly evolve without fixed structures.
Eső & Szentes (2017) show that in dynamic contracting, information asymmetry disappears when intelligence is abundant—exactly what happens when AI democratizes knowledge.
This paper presents a new economic framework for understanding AI-driven disruption:
Zero-cost execution shifts competitive advantage from cost to differentiation.
Firms no longer scale through efficiency, but through AI-enabled strategic design.
The ability to generate novel business models at zero cost leads to an explosion of economic value.
This section formalizes the economic foundations of AI-driven zero-cost innovation. It lays out core assumptions, key economic variables, and theoretical propositions that describe how LLMs reshape business models by eliminating execution costs and shifting competition towards strategic differentiation.
Traditional economic models assume that production and knowledge creation require significant costs (Arrow, 1962; Romer, 1990). The defining feature of LLMs and AI-native businesses is that they destroy these cost structures.
Marginal Cost of Intelligence → Approaching Zero
Once an LLM is trained, the cost of generating intelligence, strategy, or execution is effectively zero.
This collapses traditional cost-plus pricing models, disrupting firms that monetize knowledge-based services.
Zero-Cost Innovation → Infinite Business Model Iteration
AI allows firms to test and refine business models, pricing structures, and market strategies instantly.
This eliminates the traditional R&D constraints that once limited firms.
Competitive Pressure Shifts from Efficiency to Differentiation
In traditional markets, firms competed on cost efficiency (Porter, 1985).
With execution becoming free, differentiation through unique value creation becomes the only viable advantage.
To formally analyze AI-driven business disruption, we define four key assumptions:
In traditional firms, knowledge is rivalrous (e.g., an expert consultant cannot serve multiple clients simultaneously).
AI removes this constraint—LLMs generate unlimited intelligence without additional cost per unit.
This aligns with non-rival goods in economic theory (Samuelson, 1954), but with an even greater impact due to real-time adaptability.
AI allows companies to generate, test, and refine strategies at zero cost and infinite speed.
Traditional firms rely on trial-and-error and market research, while AI firms can run simulations in real-time.
Firms no longer gain an edge by cutting costs—they gain it by creating unique, AI-powered business models that are impossible to replicate easily.
AI-native businesses must focus on how value is created, not just how it is executed.
Unlike traditional firms with fixed business structures, AI-native companies constantly evolve based on real-time data and AI-driven insights.
This means static market positioning disappears—firms must be fluid, adaptable, and continuously reinventing themselves.
Based on these assumptions, we derive the following formal economic propositions that describe the behavior of AI-native businesses and their impact on competitive markets.
Let C(K) be the cost of producing K units of knowledge work.
In traditional firms, C(K) > 0 due to labor, expertise, and execution costs.
With AI, C(K) → 0 as AI replaces manual execution and strategic analysis.
Knowledge-based firms that previously relied on charging per unit of expertise face immediate disruption as AI commoditizes their output.
Industries like consulting, legal services, and research-based firms collapse unless they shift towards AI-powered differentiation.
Blue Ocean Strategy (Kim & Mauborgne, 2005) defines a unique market space where competition is irrelevant.
Traditionally, firms needed R&D, capital, and time to create such markets.
With AI, firms can generate, test, and refine new business models instantly, creating endless differentiation opportunities.
Market structures will become fluid and dynamic, as companies can pivot into new niches at near-zero cost.
Static industry boundaries disappear, leading to constant reconfiguration of business ecosystems.
Traditional economic models assume firms compete on cost efficiency (Porter, 1985).
AI-driven firms operate at zero marginal cost, making price competition unsustainable.
The only remaining competitive factor is how unique and strategically differentiated a firm’s offerings are.
Business model agility becomes the primary determinant of success.
Firms must operate as self-optimizing intelligence engines that continuously refine their strategic positioning.
Democratization of Business Creation
Anyone with AI access can create, launch, and iterate a business model without significant capital investment.
This reduces barriers to entry and creates an explosion of entrepreneurial activity.
Extreme Competitive Volatility
Traditional firms struggle because their advantages are static while AI-driven firms evolve dynamically.
The concept of “sustainable competitive advantage” (Barney, 1991) becomes obsolete—advantage is now fleeting and continuously redefined.
Continuous Industry Disruption
Unlike past technological shifts that disrupted one industry at a time, AI-native firms disrupt all industries simultaneously.
Every market becomes a fluid, adaptive ecosystem where businesses are constantly redesigning themselves.
This section establishes the core economic logic behind AI-driven zero-cost innovation:
When intelligence is infinite and free, execution-based business models collapse.
Firms must differentiate through continuous strategic innovation, not cost efficiency.
Industry boundaries and market structures become fluid, driven by AI-powered optimization.
Having established the theoretical foundations of infinite intelligence and zero-cost innovation, this section explores the real-world strategic and economic consequences. The disappearance of marginal execution costs is not just an efficiency gain—it fundamentally reshapes competitive dynamics, industry structures, and economic value creation.
In traditional economics, firms sustain competitive advantage through moats—barriers that protect them from rivals. These moats have historically included:
Cost Leadership (Porter, 1985) → Competitive advantage through economies of scale and operational efficiency.
Brand & Intellectual Property (Barney, 1991) → Firms differentiate through brand loyalty, patents, and proprietary knowledge.
Network Effects (Rochet & Tirole, 2003) → Platforms dominate by locking in users and reinforcing their market position.
AI fundamentally erodes these moats:
Cost Leadership Moat Dies: When AI-driven automation removes labor costs, cost-based competition collapses. No one can maintain an advantage when execution is free.
IP and Knowledge Moats Erode: AI can generate, summarize, and recombine knowledge instantly, reducing the defensibility of proprietary expertise.
Network Effects Are Challenged: AI reduces switching costs by enabling frictionless automation and seamless integrations, allowing users to migrate platforms with zero learning curve.
Example:
Consulting firms used to have an advantage based on deep expertise and high-cost advisory services. Now, AI can generate the same insights instantly at near-zero cost, forcing them to compete on strategy and brand rather than expertise alone.
Traditional firms operate under fixed business models that require:
Long R&D cycles to develop new offerings.
Capital-intensive scaling to expand market presence.
Rigid operational structures to maintain competitive advantage.
AI-native firms, in contrast, function as self-optimizing intelligence networks:
Instant business model iteration: AI enables firms to continuously test and refine offerings in real time.
Real-time customer adaptation: AI-driven personalization creates adaptive pricing, dynamic product-market fit, and frictionless onboarding.
Modular and fluid organizational structures: Instead of rigid hierarchies, AI-native firms operate as distributed, algorithm-driven entities.
With AI-native enterprises, the concept of a static business model disappears. Instead:
Business models become self-adaptive algorithms that adjust based on real-time market feedback.
Companies constantly refine their offerings without the constraints of physical production or human decision-making bottlenecks.
Example:
Traditional SaaS (Software-as-a-Service) companies operate on fixed subscription models.
AI-native SaaS companies, however, will move towards intelligent, real-time value-based pricing, dynamically adjusting their revenue models based on user engagement, intent, and demand elasticity.
When execution becomes free and frictionless, the primary constraint on innovation is removed. This creates a paradigm shift in entrepreneurship:
Pre-AI Era: Building a company required capital, labor, and operational complexity.
AI-Era: Anyone can launch an autonomous business with zero-cost innovation cycles and real-time strategic adaptation.
AI enables instant business model design, automated market validation, and dynamic repositioning.
Micro-entrepreneurship explodes as individuals can create AI-powered businesses with no upfront investment.
In traditional markets, visionary founders held an edge due to unique insights and execution capabilities.
AI flattens this advantage—anyone with access to LLMs can generate, test, and optimize business models instantly.
Example:
Instead of a venture-funded startup spending years in R&D, an individual can generate, refine, and launch AI-powered digital businesses in minutes, scaling purely through automated intelligence.
Key Insight:
Entrepreneurial barriers collapse, leading to an explosion of AI-generated micro-enterprises.
When execution is automated, human work shifts toward strategic architecture.
The new economy is defined by who can design the most compelling, high-value AI-driven business models.
Firms no longer compete on operational capacity, but on their ability to continuously craft differentiated strategies.
Traditional firms plan strategies annually or quarterly.
AI-native firms adjust in real-time, constantly repositioning themselves based on real-time data and AI-driven insights.
While AI automates execution, human ingenuity is still essential.
The highest-value roles will be those who can craft business models, market narratives, and value propositions that AI cannot generate on its own.
AI-native firms may create winner-take-all dynamics where companies with the best data dominate industries.
Regulators must rethink antitrust models, as traditional measures of market power become obsolete.
The automation of knowledge work will reshape job markets, requiring policymakers to redefine workforce development strategies.
There will be a massive shift toward high-level strategic roles, but traditional white-collar labor markets will shrink.
AI can instantly generate deceptive, manipulative, or exploitative business models, raising concerns about consumer protection and regulatory oversight.
Governments must develop frameworks for ensuring AI-driven business ethics.
This section outlines the fundamental economic shift brought by AI-driven intelligence abundance, the elimination of execution costs, and how this redefines business models, competitive dynamics, and value creation.
For centuries, economic models have been built on the assumption that labor, knowledge, and capital are scarce resources (Arrow, 1962; Romer, 1990). Firms gained competitive advantage by efficiently managing these constraints—whether through cost optimization, labor productivity, or knowledge specialization.
LLMs fundamentally break this scarcity assumption by making high-level intelligence an abundant, zero-marginal cost input. Unlike past technological revolutions, which increased labor productivity, AI eliminates the need for labor in execution entirely. This leads to:
The collapse of traditional business constraints—what once required capital, expertise, and teams can now be automated by AI.
The death of incremental labor costs—knowledge work is no longer a scarce asset but an instantly available, scalable input.
A fundamental restructuring of business economics—value creation moves from operational efficiency to continuous strategic differentiation.
The rise of infinite intelligence restructures the core mechanisms of business and competition:
Traditional Economy: Hiring experts, consultants, or R&D teams was expensive and slow.
AI Economy: Any firm, regardless of size, can generate instant high-level intelligence for near zero cost.
Impact:
Industries that monetize knowledge-based expertise (consulting, legal, financial analysis) face instant disruption.
Cost-plus pricing models collapse because intelligence can no longer be sold as a scarce good.
Traditional Economy: Product development, strategic planning, and market entry take months or years due to human-driven R&D and testing cycles.
AI Economy: AI enables instant ideation, testing, and iteration, allowing firms to rapidly launch and refine business models in real time.
Impact:
Traditional barriers to entry disappear, as startups can match or exceed incumbents’ capabilities instantly.
Firms that rely on long-term product cycles (pharma, hardware, deep-tech R&D) must rethink their business models.
Traditional Economy: Once a product is launched, firms must wait for market feedback, adapt slowly, and retool operations.
AI Economy: AI-driven businesses operate as self-optimizing intelligence engines, where:
Products evolve continuously based on real-time customer interactions.
AI dynamically tests new offerings, pricing models, and business strategies without human intervention.
Impact:
The traditional concept of a "finished product" disappears—everything is in a state of perpetual adaptation.
Companies must compete on agility, as AI-native firms can disrupt them overnight by out-iterating them in real time.
To formalize how AI-native firms function, we establish three foundational assumptions that define their structure and strategy.
In traditional business models, intelligence is scarce and costly (requiring human expertise, training, and knowledge transfer).
In the AI-native economy, intelligence is non-rival—once an AI model is trained, its insights can be instantly and infinitely replicated at zero cost.
Implications:
Knowledge-based industries (law, consulting, finance, strategy) collapse unless they build AI-driven differentiation.
The role of competitive advantage shifts from knowledge accumulation to real-time business model experimentation.
AI enables mass democratization of business creation, as individuals gain access to world-class intelligence tools without needing capital or specialized expertise.
Historically, companies gained an advantage by hiring better workers, building better factories, or optimizing supply chains.
AI eliminates execution as a bottleneck, meaning firms no longer compete on who can produce more efficiently—instead, they compete on who can architect the best system of value creation.
Implications:
Economies of scale become irrelevant—small AI-native firms can match or exceed the output of large corporations.
Product-based businesses must rethink their value proposition, as AI-native firms can replicate and improve upon existing products in real time.
Firms must compete on continuous reinvention, as static business models become obsolete in an adaptive AI-driven world.
Since execution is fully automated, human labor is no longer valuable for repetitive or operational tasks.
The only remaining competitive advantage is the ability to design and orchestrate AI-driven value creation models.
Implications:
Strategic vision becomes the core differentiator—firms must focus on continuously crafting differentiated, high-value business models rather than optimizing execution.
Industries centered around executional labor shrink, while new opportunities emerge for AI-driven strategic innovation.
The concept of employment changes—instead of managing operations, humans design AI-powered businesses that self-optimize.
Key Takeaway:
AI eliminates execution-based moats, forcing firms to compete on business model reinvention and differentiation.
We are moving from an era of efficient production to an era of infinite business model iteration.
As AI-driven automation eliminates execution costs, the foundations of traditional competition collapse. The ability to produce efficiently was once the core differentiator, but when execution becomes free and instant, companies must instead compete on continuous strategic reinvention. This shift leads to the death of cost-based competition, the rise of AI as an infinite business architect, and the emergence of the Architect Economy.
For centuries, businesses gained competitive advantage through cost efficiency—whether by reducing labor costs, optimizing supply chains, or achieving economies of scale (Porter, 1985). However, AI disrupts this paradigm by removing execution as a constraint, making cost-based advantages obsolete.
Traditional Model: Compete by cutting costs, improving operational efficiency, and increasing scale.
AI-Native Model: Instantly build and iterate new business models without any execution concerns.
Businesses that rely solely on cost efficiency will become obsolete as AI-powered firms can create, test, and optimize unique value propositions instantly.
The biggest impact of AI is not just automation—it is its ability to act as an infinite business architect that designs and optimizes business models at near-zero cost.
Traditional firms required teams of strategists, analysts, and consultants to design business models, research markets, and optimize operations. LLMs remove this bottleneck by allowing a single individual to generate and deploy an optimized business strategy instantly.
An individual can now:
Use AI to identify gaps in the market → AI models analyze consumer demand, trends, and competitor weaknesses.
Generate product designs, pricing models, and marketing strategies → AI can craft a full business plan in seconds.
Deploy a fully optimized business—without any upfront capital → AI automates the entire launch process, from website creation to targeted advertising.
Impact:
No expertise or large teams required → Business creation becomes as simple as running AI queries.
Barriers to entry vanish → A global explosion of AI-powered micro-entrepreneurs reshapes traditional industries.
Traditional firms lose their knowledge advantage → AI democratizes business strategy and decision-making.
As AI eliminates execution constraints, the role of human labor shifts from manual execution to strategic design. This marks the birth of the Architect Economy, where everyone is a creator, strategist, and business model innovator.
From manual execution → To infinite ideation and real-time strategic pivots
From production-based economies → To business model innovation as the primary value driver
From workforce-based scaling → To AI-driven, self-optimizing companies
The winners of the AI-driven economy will be those who architect and adapt business models in real-time, rather than those who focus on cost efficiency or static market positioning.
The rise of LLMs and AI-driven automation has triggered an explosion of value creation by fundamentally changing how businesses operate, compete, and innovate. With execution costs approaching zero, the basis of competition shifts from efficiency to strategic differentiation, making hyper-personalized, AI-driven business models the new standard.
This section explores how AI-native firms redefine business models, enable instant Blue Ocean Strategy creation, and eliminate traditional industry boundaries.
Historically, businesses gained competitive advantage through efficiency—by cutting costs, improving logistics, and optimizing speed. However, in a world where AI automates execution at zero cost, efficiency is no longer a viable differentiator.
Before AI: Firms competed on cost, speed, and logistics (lean manufacturing, supply chain optimization, economies of scale).
Now: Firms compete on uniqueness, conceptual design, and strategic differentiation.
SaaS (Software-as-a-Service) Companies:
Before: Traditional SaaS companies sold fixed-feature software products with subscription-based pricing.
Now: AI-driven SaaS hyper-personalizes software in real time, adapting features dynamically based on user behavior and context.
Consulting Firms:
Before: Strategy consulting relied on large teams of analysts conducting market research and creating reports.
Now: AI-native firms operate as autonomous strategic advisors, delivering instant, AI-generated business strategies and market insights.
Marketplaces and E-Commerce:
Before: Marketplaces competed primarily on pricing and supply-chain optimization.
Now: AI-driven platforms eliminate price-based competition by using real-time demand prediction and personalized recommendations, shifting power to experience-driven business models.
Competitive advantage is no longer about cost efficiency—it’s about continuous, AI-driven reinvention.
Traditionally, businesses that pursued Blue Ocean Strategy—creating entirely new, uncontested markets—faced significant barriers in terms of capital, R&D, and execution complexity (Kim & Mauborgne, 2005).
With AI, these barriers disappear:
AI enables instant, dynamic strategy creation.
AI allows businesses to reposition themselves in real-time, adapting to competitive shifts instantly.
Anyone, from solo entrepreneurs to large enterprises, can create hyper-differentiated businesses instantly.
AI-driven business models do not adhere to fixed industry categories—they continuously evolve, merging functionalities from multiple sectors to create adaptive, hybrid businesses.
AI enables businesses to expand beyond their core industry at zero cost, integrating new capabilities instantly.
Companies no longer belong to single, well-defined industries—they operate fluidly across multiple sectors based on AI-driven intelligence.
Logistics Becomes Fintech:
A logistics company integrates AI-driven financial modeling, offering automated supply chain financing and predictive payment structures, effectively merging logistics with fintech.
Media Becomes Education:
A media company transforms into an AI-powered education platform, using AI-generated courses, personalized learning, and real-time content adaptation.
Before AI: Companies were confined to one primary industry, requiring capital and expertise to expand into new markets.
Now: AI enables instant cross-industry expansion, turning every company into a fluid, adaptive entity that continuously redefines itself.
Industry boundaries no longer define business capabilities—adaptability and AI-driven reinvention do.
While AI-driven zero-cost innovation presents immense opportunities, it also introduces economic, strategic, and ethical challenges that require deeper analysis. This section explores the key limitations of AI-native business models and proposes areas for further research to address unresolved questions.
Despite the transformative potential of AI-native firms, several structural and economic constraints remain:
AI-native firms rely on large-scale data access to train models and refine business strategies.
Dominant AI platforms (e.g., OpenAI, Google, Meta) control access to the most advanced models, creating data monopolies.
Barrier to Entry Concern: If AI-native firms require proprietary datasets, innovation could become concentrated among a few players, reducing competitive dynamism.
When AI generates strategies, business models, and content, there is a risk of convergence—where outputs become indistinguishable.
If everyone accesses the same AI-driven insights, differentiation becomes difficult.
Paradox: AI enables mass innovation but could lead to strategic uniformity, requiring firms to develop new mechanisms for maintaining uniqueness.
AI-native firms operate without deep capital reserves, human expertise, or physical assets.
Risk: If AI models fail or regulations change, entire AI-dependent business ecosystems could collapse.
Example: A business that automates 100% of its operations through AI is vulnerable if an LLM API pricing shift or service outage disrupts its operations.
AI-native business models currently depend on human input for high-level strategic design.
The line between AI-augmented decision-making (human + AI) and full AI autonomy (self-running firms) remains unclear.
Future firms will need to balance human creative oversight with AI-driven adaptability to avoid unintended consequences.
Several unanswered questions emerge from the rise of AI-native firms:
Traditional economic models assume firms optimize business models over time based on learning curves and market positioning.
AI-native firms iterate strategies instantly, collapsing the traditional business learning cycle.
Open Question: What happens when every company continuously experiments and optimizes its business model in real time?
Classical economic models assume firms reach stable equilibrium points through competitive interactions.
AI-driven firms operate in a state of constant, adaptive flux, challenging the notion of market stability.
Open Question: Will AI-driven competition lead to chaotic, non-equilibrium markets where firms constantly shift strategic positioning?
AI-driven automation eliminates knowledge work bottlenecks, shifting human labor toward strategic architecture.
However, as AI advances, even high-level strategic roles could become automated.
Open Question: What happens when AI can design and adapt business models without human intervention?
AI-driven firms operate with real-time adaptability, evading traditional regulatory frameworks.
Governments struggle to regulate AI-native firms that do not fit traditional corporate structures.
Open Question: Should governments regulate AI-native firms based on adaptive intelligence-driven operations rather than fixed business structures?
The rise of AI-driven infinite intelligence marks a fundamental economic shift—not just an improvement in business operations but the total redefinition of how businesses function, compete, and create value.
This paper has demonstrated that AI does not merely enhance traditional business models—it renders them obsolete.
Execution is commoditized; strategic design is the new competitive advantage.
AI eliminates execution as a bottleneck, making efficiency-based competition irrelevant.
The firms that thrive will be those that continuously reinvent and differentiate themselves, rather than optimize production.
Anyone can create high-value, unique business models at near-zero cost.
AI democratizes entrepreneurship, allowing individuals to launch sophisticated businesses without capital, technical skills, or operational infrastructure.
Traditional barriers to market entry and competitive differentiation collapse, creating an explosion of AI-driven micro-enterprises and adaptive firms.
Business models become fluid and self-evolving.
AI enables continuous iteration, where firms constantly adjust their strategies, offerings, and positioning in response to real-time market conditions.
This challenges existing economic theories of market equilibrium and firm stability, as AI-native businesses operate in a perpetual state of adaptation.
While AI-native business models introduce unprecedented opportunities, they also raise new theoretical, economic, and strategic questions that demand further research.
AI-native firms may not require human intervention—instead, they function as self-optimizing intelligence systems that autonomously refine their own strategies, products, and market positioning.
Key Question: What happens when businesses become fully autonomous, evolving without human leadership?
Potential Impact: The rise of self-improving digital corporations, fundamentally altering firm dynamics and competitive markets.
AI enables businesses without employees—firms that operate solely on algorithms, automation, and AI-driven decision-making.
Key Question: How should we define and regulate corporations that have no human workers, managers, or traditional leadership structures?
Potential Impact:
The emergence of fully AI-driven companies that operate independently of traditional human governance.
The legal, ethical, and regulatory challenges of AI-native corporate entities.
AI can generate infinite strategic possibilities, business models, and market opportunities—but human creativity remains a key differentiator.
Key Question: As AI automates design, execution, and strategic thinking, what remains uniquely human in business creation?
Potential Impact:
A redefinition of human labor—shifting from knowledge execution to abstract creativity, judgment, and high-level ideation.
The emergence of new cognitive frontiers, where human-AI collaboration becomes the dominant mode of value creation.
We are entering an economic era where business models are no longer static structures—they are fluid, continuously evolving entities shaped by AI-driven intelligence.
The central challenge of the post-scarcity, AI-driven economy will not be how to produce things efficiently—it will be how to architect, differentiate, and continuously adapt value creation models in an infinitely intelligent world.
The future belongs to those who do not just use AI to improve their businesses—but to those who redefine what a business can be.