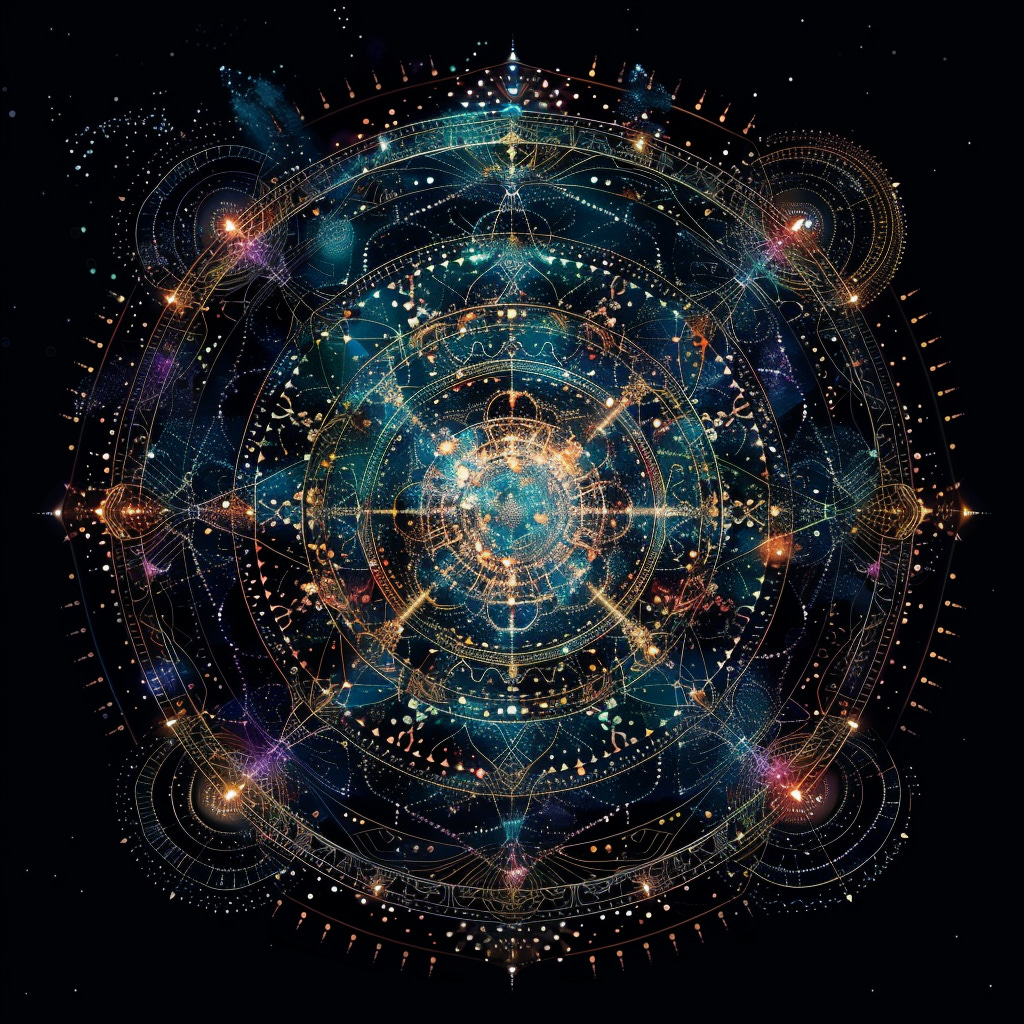
April 11, 2025
Shaping a national AI strategy through the lens of layered architecture is not a matter of compiling initiatives—it is an act of strategic systems design. It begins with recognizing that AI is not a sector but a civilizational infrastructure, touching everything from language to logistics, from diplomacy to disease response. A true strategy cannot be flat; it must be multi-dimensional, with layers that build upon one another and levers that stretch across domains. A nation cannot afford to focus only on innovation without infrastructure, or on deployment without values. It must align technical ambition with societal need and geopolitical foresight.
To do this, we must first recognize the Creative Layer as the generative nucleus. This is where AI capabilities are invented, built, and productized—where research, systems engineering, and business model experimentation converge. For nations with scientific depth and technical maturity, this is the sharp edge of competition. But even nations without frontier R&D capacity can thrive here by focusing on modular system design, verticalized product creation, and AI-native entrepreneurship. The creative layer is where AI becomes expressive, where the economy begins to reorganize around new patterns of automation and insight.
Yet creation is not enough without Strategic force projection. Talent must be attracted, not just grown. Norms must be shaped, not just consumed. At this layer, nations extend their influence outward—not through military or monetary might, but through ideas, institutions, and interoperability. Strategic tools like AI diplomacy, global talent attraction, and interdisciplinary startup ecosystems become essential. These mechanisms ensure a nation's values and capacities are not just operational domestically, but relevant globally, helping shape how AI unfolds beyond its borders.
The Supportive Layer is where ambitions either scale or suffocate. Data, compute, and education are not optional—they are the oxygen of any AI ecosystem. A strategy that neglects this layer will fragment, regardless of how impressive its labs or startups may be. Supportive infrastructure transforms AI from an elite capability into a national utility. When compute is sovereign, data is governed, and universities are AI-native, the system becomes resilient, scalable, and democratized. The AI-capable state emerges from these deep investments—not from slogans, but from scaffolding.
Then comes the Applied Layer—where everything must land. This is the ultimate test of any strategy: can AI make the state more agile, the economy more inclusive, and the society more intelligent? From smart agriculture to adaptive education, from crisis response to judicial transparency, this layer is about embedding AI into the daily functioning of the nation. Here, AI becomes a policy tool, a public service amplifier, and a civilization-scale feedback loop. The applied layer is not the end of the process—it is its validation.
A national AI strategy built on this layered foundation is not a laundry list—it is a living architecture. Each layer feeds the others. Each requires the others. Strategy emerges not from imitation of superpowers, but from identifying where the nation sits within these layers, where it can lead, where it must support, and where it can deploy. This approach allows every country—not just the technologically dominant—to find its sovereign path through the AI age. It turns scattered initiatives into a coherent machine, and reactive policy into anticipatory governance.
Where intelligence is not just used—but made
The Creative Layer is the generative nucleus of national AI capacity. This is where nations move from consumers to producers of intelligence. It includes the creation of new algorithms, architectures, products, and economic logics. Countries strong in this layer define their own technological trajectory rather than importing it. It demands scientific depth, engineering talent, and entrepreneurial elasticity.
This is where foundational capabilities are built and combined into systems, where AI is embedded into software and physical infrastructure, and where entirely new businesses and industries emerge.
Core Components:
Core AI Research & Model Development – Inventing algorithms, architectures, and training paradigms.
Component-Based System Architecture – Building modular AI platforms using open-source or commercial models.
AI-Centric Product Engineering – Turning capabilities into usable tools and customer-facing software.
AI-Native Business Model Innovation – Creating companies that only exist because AI makes them possible.
Enterprise Productivity Amplification – Injecting AI into the decision-making and operational layers of existing organizations.
This layer is critical not just for innovation but for economic power projection—nations strong here export not only software but also new modalities of work, insight, and automation.
The geopolitical and systemic force multipliers of AI capacity
The Strategic Layer is the global positioning system of national AI policy. It doesn’t create algorithms or products—but it determines who gets to participate, who sets the rules, and how talent and capital flow across borders. This layer establishes the structural leverage needed to either dominate, shape, or harmonize with the global AI economy.
Strategic levers allow a nation to:
Pull in international expertise and resources
Seed entire sectors through interdisciplinary startup ecosystems
Export its regulatory and ethical values into the international AI order
Core Components:
Interdisciplinary Startup Creation & Support – Fusing domain depth with AI capability to launch high-impact companies.
Global Talent Attraction – Drawing in the world’s top researchers, engineers, and founders.
AI Diplomacy – Exporting governance frameworks, shaping global standards, and advancing values in international alliances.
Where the Creative layer generates what AI is, the Strategic layer determines who controls the context in which that AI will operate—intellectually, commercially, and politically.
The infrastructural and educational backbone of national AI capacity
The Supportive Layer constitutes the non-negotiable substrate beneath AI capability. It doesn’t innovate or regulate—but it enables both. This layer supplies the fuel, scaffolding, and distribution channels through which ideas become models, and models become tools. Without it, everything else becomes bottlenecked, brittle, or externally dependent.
It encompasses data, compute, education, training, and testbeds—the “hard and soft infrastructure” required to activate, scale, and govern AI systems nationally.
Core Components:
Data Ecosystem Creation – Building open, secure, and privacy-compliant data lakes and repositories.
Compute Infrastructure – Providing sovereign, equitable, and sustainable access to training and inference capacity.
University Education Augmentation – Reengineering higher education to produce AI-fluent professionals across all domains.
Workforce AI Training – Upskilling the broad workforce with modular AI fluency and practical literacy.
AI Deployment Testbeds – Providing controlled environments to trial AI systems in sensitive or mission-critical domains.
This is the layer that determines whether a nation's AI strategy is scalable, sustainable, and inclusive—or constrained to isolated labs and startup clusters.
Where AI becomes a national function
The Applied Layer is where AI becomes embedded in the real world—in the state, in institutions, in infrastructure. This layer is the operational expression of AI capacity. It transforms AI from a research field or an economic catalyst into a public instrument, a governance enhancer, and a civilizational amplifier.
What defines this layer is not invention but deployment at scale—across sectors, services, systems, and crises.
Core Components:
Sectoral AI Orchestration – Optimizing agriculture, energy, logistics, and other strategic verticals.
Public Health & Biosecurity AI – Enhancing diagnostics, outbreak detection, and medical R&D.
Legal and Judicial System Augmentation – Supporting casework, access to justice, and regulatory enforcement.
Crisis & Disaster Response – Real-time perception and coordination during national emergencies.
Education System Personalization – Adaptive learning and national tutoring copilots.
Civic & Governmental AI Deployment – Using AI to make governments more efficient, transparent, and responsive.
Scientific Research Augmentation – AI-accelerated discovery in biology, climate science, physics, and beyond.
AI for Global Challenges – Climate, inequality, pandemics—planetary problems solved by planetary intelligence.
This layer is where AI stops being a sector and becomes a national nervous system—used not just to automate tasks, but to amplify the intelligence of the state and society itself.
This is the epistemic heart of national AI power. Core AI research drives the creation of original learning architectures, new training paradigms, and foundation models with general-purpose capabilities. Nations active in this space don't just use AI—they shape what AI is. This is the layer where paradigm shifts happen.
To operate effectively at this level, a nation must have:
Elite Research Institutions
Universities, AI labs, and think tanks producing frontier papers and open models.
Sovereign Compute Infrastructure
Access to massive-scale GPU/TPU arrays and exascale HPC clusters.
AI Research Talent Density
A critical mass of top-tier PhDs, postdocs, and principal investigators in machine learning, optimization, cognitive science, etc.
Unrestricted Access to High-Quality Data
Multimodal, diverse, large-scale datasets across domains.
Freedom to Explore High-Risk Problems
Funding schemes that support 10+ year horizon research, moonshots, and general intelligence work.
Open Collaboration Networks
Participation in global peer networks like NeurIPS, ICML, ICLR, and arXiv leadership.
Development of foundational models: LLMs, diffusion models, multimodal agents.
Generation of new algorithms, architectures, and training methods.
Creation of benchmark datasets and evaluation frameworks for global use.
Ability to release or commercialize open-source models (e.g. LLaMA, Falcon).
🇺🇸 OpenAI, Anthropic, DeepMind (UK/US), Meta AI Research: Training frontier models (GPT-4, Claude, Gemini).
🇨🇦 MILA (Montreal Institute for Learning Algorithms): Pioneered deep learning with Yoshua Bengio.
🇬🇧 Alan Turing Institute: Combines national compute, academic power, and ethical foresight.
🇫🇷 Inria & CNRS: Core research in machine reasoning, symbolic logic, and verification.
🇨🇳 Beijing Academy of AI & Tsinghua University: Pangu, WuDao, and GLM large-scale foundation models.
This layer makes AI operational. It doesn’t invent algorithms—but it orchestrates them. Component-based system architecture allows nations to build domain-specific AI stacks, tailored to local industries, institutions, and languages. It is where practical sovereignty is exercised, without requiring foundational model development.
To activate this layer, a nation needs:
Strong Software Engineering Workforce
Devs who can integrate models with backend systems, UI, data pipelines, and APIs.
Access to Foundation Models & APIs
Through open-source (e.g. LLaMA, Mistral), commercial (OpenAI, Cohere), or licensed partnerships.
Domain-Specific Knowledge Graphs & Ontologies
Structured domain expertise to contextualize model behavior (e.g., legal, medical, logistics).
Secure, Modular Infrastructure
Containerization, inference orchestration, monitoring, and model serving pipelines.
Regulatory Navigation Capability
Legal frameworks for deploying AI safely in high-stakes domains.
Product–Policy–System Integrators
Engineers who work fluently across software, organizational workflows, and policy interfaces.
Assembly of intelligent systems using models for perception, language, reasoning, and planning.
Pipeline architectures that fuse ML inference with real-time data and human feedback loops.
Modular designs that allow for safe upgradability and domain transfer.
Interfaces for human-in-the-loop interaction, auditability, and control.
🇩🇪 Siemens + Fraunhofer Institute: Building smart manufacturing AI layers using modular components.
🇮🇱 Israel’s healthtech sector: Componentized AI systems for diagnosis, triage, and digital pathology.
🇸🇬 AI Singapore’s 100E program: Partners industry with system architects to build deployable sectoral solutions.
🇫🇮 Valohai: Provides infrastructure to orchestrate and monitor complex componentized AI workflows.
🇺🇸 Palantir + Databricks: Offer platformized AI system orchestration at scale across government and enterprise.
This is where AI meets usability. Even the most powerful model is useless without being embedded in coherent, elegant, and secure products. This layer transforms raw model outputs into structured, accessible, value-generating tools—for enterprises, consumers, and public services alike.
To lead in this domain, a country needs:
Human-Centered Design Culture
UX/UI excellence, HCI talent, and empathy-driven product development.
Product-Minded Engineers
Developers who think in use cases, not just features—who ship, test, and iterate rapidly.
Access to MLOps & LLMOps Tooling
Tools like LangChain, Haystack, RAG pipelines, vector DBs, prompt tuning, etc.
Startup Ecosystem or Innovation Teams
Small, agile orgs that experiment with speed and failure tolerance.
Legal & Compliance Frameworks
Especially in regulated sectors like finance, healthcare, or defense.
Localization Infrastructure
Language, culture, and domain adaptation for local contexts.
Development of apps, platforms, and interfaces powered by generative or analytical models.
Integration of AI outputs into structured workflows, dashboards, and decision systems.
Emphasis on feedback loops, explainability, and responsive interaction.
Agile iteration and product-market fit discovery in new AI-native categories.
🇳🇱 Picnic (Netherlands): AI-based grocery fulfillment with complex backend orchestration.
🇸🇬 MindFi: AI-enhanced mental health platform tuned for Southeast Asian cultural contexts.
🇺🇸 Notion, Replit, Tome: Exemplars of product-centric AI engineering—blending usability and capability.
🇬🇧 Synthesia: AI-generated video product with UX-optimized scripting and editing layers.
🇪🇪 Veriff: AI-enhanced identity verification product rooted in privacy-respecting product design.
This layer represents the entrepreneurial frontier of the AI economy. It’s not about building AI systems—it’s about building companies that would be impossible without AI. AI-native businesses unlock entirely new markets: problems that were too niche, too expensive, too complex, or too dynamic to be addressed by traditional methods.
This layer also enables non-traditional founders (e.g., researchers, domain experts, solo builders) to launch startups by outsourcing cognitive labor to models. It democratizes the founding of companies, not just the building of tools.
Startup Culture & Entrepreneurial Tolerance
Ecosystems that embrace experimentation, failure, and nonlinear risk.
Access to Capital with a High-Risk Appetite
Early-stage funding for unproven ideas—especially for AI-embedded services.
Builder–Founder Talent Pool
Technically literate individuals with both domain insight and product intuition.
AI-First Toolchains
Infrastructure to quickly prototype with LLMs, agents, vector search, and APIs.
Founder-Friendly AI Access
Preferably public models, API credits, open weights, and licensing flexibility.
Regulatory Clarity
Particularly for data use, privacy, and liability in AI-generated outputs.
Business models that rely on intelligence as a service—where a model performs 80% of a traditionally human task.
Ultra-lean operational structures, often 1–10 person teams running multi-million-dollar products.
Startups that serve fragmented, previously unservable sectors: micro-consulting, local governance tools, rare disease research, etc.
Dynamic products that improve over time via user–model feedback loops.
🇺🇸 LegalMation: AI generates litigation documents—selling legal output, not just tooling.
🇸🇬 Hypotenuse AI: One-person e-commerce content generation startup with global clients.
🇫🇷 Hugging Face: Not just an AI lab, but a business ecosystem built around model hosting, benchmarking, and democratized access.
🇦🇪 Kalima Systems: Modular AI + IoT platform aimed at hyper-specialized industrial use cases.
This is where AI becomes a force multiplier inside existing businesses. It’s not a new business model—it’s a new metabolism. AI transforms how enterprises:
Explore R&D hypotheses,
Serve customers,
Make decisions,
Conduct operations.
The organizations that master this layer don’t just become more efficient—they become more strategically intelligent. They replace bottlenecks with feedback loops and manual labor with cognition-on-demand.
Digitally Mature Enterprises
Cloud adoption, data pipelines, modular workflows already in place.
AI-Literate Executives & Managers
Leaders who understand where AI fits—not just technically, but culturally.
Access to Bespoke AI Integrators
Consultants or internal teams that can adapt foundation models to enterprise contexts.
Trust Infrastructure
Data governance, auditability, and risk mitigation protocols.
Permissionless Experimentation
Sandboxes inside the organization for bottom-up AI deployment.
Data Maturity
Structured, tagged, and accessible internal data to power custom workflows.
Copilot layers built into CRM, ERP, internal analytics, HR, and procurement systems.
Domain-specific generative models trained on proprietary workflows (e.g., pharma R&D, logistics, law).
Decision augmentation systems that allow executives to simulate scenarios or explore strategic options.
“Second-brain” setups for departments—AI agents that summarize, analyze, and suggest without supervision.
🇩🇪 Bosch: Uses AI across supply chains, predictive maintenance, and smart manufacturing workflows.
🇯🇵 Mitsubishi UFJ Financial Group: Internal LLMs fine-tuned on legal, audit, and banking documentation.
🇺🇸 Salesforce Einstein & Microsoft Copilot integrations: AI fused into core enterprise software stacks.
🇩🇪 SAP: Building generative copilots into its ERP ecosystem across global clients.
🇺🇸 Morgan Stanley: Custom GPT trained on 100,000+ pages of internal knowledge for advisors.
🇫🇷 Sanofi: Internal AI assistant for R&D literature synthesis and drug repurposing.
🇸🇬 Temasek: AI-driven investment analysis and scenario forecasting.
Insight: This is where national productivity multipliers emerge—especially for countries with a large industrial or service economy.
AI’s real power lies not in technology—but in combinatorial reconfiguration. The frontier is no longer “AI startups,” but startups at the intersection of AI and something else—biology, law, construction, education, policy.
This layer determines whether a nation can translate academic edge + domain insight into market-shaping firms. It is the difference between being a consumer of AI and a generator of new economic categories.
To thrive, a nation must possess:
Cross-Disciplinary Talent Ecosystems
AI-capable founders with non-technical domain expertise.
Non-Traditional Accelerator Programs
Incubators that mix engineers, policy experts, creatives, and scientists.
Sectoral AI Vouchers + Challenge Grants
Seed support tied to solving vertical problems (e.g., climate risk, food logistics).
Academic Spinout Infrastructure
Tech transfer offices + legal tooling to productize university research.
Rapid Access to Prototyping Resources
Public compute credits, open datasets, pre-built model APIs, sandbox regulations.
Multidisciplinary founder teams solving complex social or industrial problems
Mission-driven VC funds that prioritize AI + X convergence
Interdisciplinary demo days, challenge prizes, civic tech sandboxes
Embedded research engineers in public health, education, climate, etc.
🇺🇸 NSF Convergence Accelerators: $10M+ grants for AI startups working in health, equity, environment
🇨🇦 CIFAR AI Chairs: Co-lead ventures with social and scientific integration
🇪🇺 Horizon Europe’s Pathfinder Program: Deeptech AI spinouts for climate, energy, and food security
🇫🇷 La French Tech - Health & Deeptech Tracks: Interdisciplinary startup pipelines seeded with state capital
Talent is no longer “mobile”—it’s liquid. Nations that can magnetize the world’s best AI builders and thinkers become gravitational nodes of innovation, even without homegrown giants.
In a world of distributed AI tools, who you attract determines what you build. And who you retain shapes your long-term epistemic sovereignty.
Talent-Accelerated Immigration Policies
Visas based on skill and portfolio, not employer sponsorship.
World-Class Research Institutions
Magnet labs and faculty chairs tied to national AI priorities.
Soft Infrastructure
Family migration support, cultural integration, multilingual services.
High-Autonomy Work Environments
Allow talent to pursue curiosity, not just KPIs.
Visible International Fellowship Programs
Well-funded, prestigious, and globally marketed.
Fast-track research and entrepreneur visas
Publicly funded “AI fellow” programs with relocation support
Global chairs at national AI labs and innovation agencies
Talent summits, intercontinental hackathons, embedded university collaborations
🇸🇬 Tech.Pass: Visa for elite AI talent, founders, and CTOs with full autonomy
🇫🇷 Talent Passport Visa + France 2030 AI Chairs: Designed to attract AI professors and lab leaders
🇺🇸 O-1 Visa + CHIPS Act Talent Initiatives: Funding foreign researchers in safety and semiconductor AI
🇩🇪 Blue Card Optimization + AI Professorships: High-tier roles offered with lab, budget, and relocation support
The most powerful nations will not merely shape AI within their borders—they will shape how it behaves everywhere.
AI Diplomacy is about value export and regulatory leverage. It defines whether your laws become global norms, whether your platforms are trusted, and whether you participate in writing the algorithmic rules of civilization.
Internal AI Governance Credibility
Transparent, accountable AI deployment at home.
Engagement in Global Governance Bodies
GPAI, OECD, UNESCO, G7, WTO AI initiatives.
Extraterritorial Regulatory Instruments
Legal frameworks (like the EU AI Act) with global enforcement mechanisms.
Multilateral AI Pacts
Cross-border research, audit, and enforcement treaties.
Capacity to Export Tools, Frameworks, and Testbeds
Toolkits like AI Verify, AI Bill of Rights, or regulatory sandboxes.
Leadership in global standard-setting (risk tiers, explainability, safety)
Normative treaties around AI in warfare, surveillance, trade
Export of AI governance infrastructure (e.g., sandbox templates, audit toolkits)
Embassies with AI advisors and digital attachés
🇪🇺 EU AI Act + TTC + Digital Services Act: EU law exported by default through extraterritoriality
🇺🇸 Blueprint for an AI Bill of Rights + OECD AI Principles + Indo-Pacific TTC
🇸🇬 Model AI Governance Framework: Adopted or adapted across ASEAN and African nations
🇨🇦 GPAI Co-Chair: Leading responsible AI + social good AI global working groups
Data is not the new oil—it’s the new gravity. Without structured, accessible, compliant, and representative datasets, AI systems become epistemically unstable and ethically dangerous. A sovereign, high-integrity data ecosystem is the minimum viable terrain on which AI competence is built.
It determines what a nation’s AI can see, understand, and reason over—across every domain from health to climate to law.
Data Stewardship Institutions
Public agencies and trusted intermediaries that manage data collection, labeling, storage, access, and ethics.
Open & Federated Data Repositories
Accessible yet controlled environments for academic, industrial, and public use.
Legal & Ethical Governance
Privacy laws, data rights, informed consent frameworks, and data sovereignty guarantees.
Data Infrastructure Tools
Metadata tagging, synthetic data engines, anonymization pipelines, version control.
Civic and Sectoral Participation
Engagement with communities and industries to source domain-rich datasets (agriculture, mobility, etc.).
National data portals with machine-readable APIs and domain tagging.
Sector-specific hubs (e.g. Health Data Hub, Climate Atlas).
Real-time data streams for urban, financial, or environmental AI.
Synthetic dataset generation frameworks to fill data deserts safely.
🇪🇺 European Data Spaces: Health, mobility, energy, and finance-focused cross-border ecosystems governed by the Data Governance Act.
🇫🇷 Health Data Hub: High-value, privacy-compliant datasets for AI researchers in health and life sciences.
🇸🇬 Trusted Data Sharing Framework: Model contracts, governance APIs, and federated access for AI innovation.
🇺🇸 NIH Bridge2AI: Biomedical datasets with embedded ethical documentation and metadata.
Without compute, data is inert, and AI is impossible. Compute infrastructure is the physical embodiment of intelligence production. It's not only about training large models—it's about enabling inference, experimentation, fine-tuning, and equal access to the AI revolution.
Nations without sovereign or shared compute become dependent, vulnerable, and technologically delayed.
High-Performance Computing Facilities
GPU/TPU clusters, data centers, exascale supercomputers optimized for ML workloads.
AI Compute Governance Framework
Equitable allocation, sustainability rules, scheduling prioritization (e.g., public research, SMEs).
Cloud-Edge Hybrid Architectures
Low-latency, secure inference environments for edge deployment (healthcare, manufacturing).
Green Compute Strategy
Liquid cooling, renewable power, carbon-aware job schedulers.
Public–Private Partnerships
Compute-sharing between government, academia, and cloud providers.
National AI cloud with usage quotas, credits, and priority tiers.
Open scheduling APIs for job submission and training pipelines.
Compute nodes across data gravity centers (universities, health agencies).
Real-time dashboards of resource utilization and energy impact.
🇫🇷 Plan France 2030: €1.5B into sovereign compute and AI-ready HPC clusters (e.g., GENCI).
🇺🇸 NAIRR (National AI Research Resource): Shared compute for public-interest research, with partnerships from Google, Microsoft, and NVIDIA.
🇯🇵 Fugaku Supercomputer: Used in AI-driven pandemic modeling and multi-scale physics simulations.
🇰🇷 Gwangju AI Cluster: AI-focused data center and compute park to serve SMEs and national R&D.
You cannot sustain AI momentum without AI-native talent. Universities are the generative engines of expertise—not only producing engineers but shaping the lawyers, designers, policymakers, doctors, and scientists who will co-create AI systems.
This layer ensures a nation doesn’t just import or upskill AI talent—but breeds a cognitively aligned generation.
Modernized Curricula Across Disciplines
AI + X programs: medicine, law, agriculture, policy, climate, ethics, etc.
Faculty Training and Development
Programs to retool professors in AI methods, tools, and teaching practices.
Intra-university AI Labs & Interdisciplinary Centers
Spaces for collaborative research, capstone design, and problem-driven learning.
Work–Study Pipelines
Apprenticeship models linking students with industry and government AI deployments.
AI Ethics & Philosophy Integration
Mandatory inclusion of safety, societal impact, and value alignment modules.
Dual-degree programs (e.g., AI + Law, AI + Biology).
National AI curriculum guidelines for technical and non-technical students.
University-based testbeds, datathons, and hackathons for applied learning.
Graduate fellowships and AI teaching assistant corps.
🇯🇵 AI/Data Science Core Curricula: Mandatory in all undergrad programs by 2025.
🇪🇺 Digital Europe Advanced Skills Program: Supports university AI reform and dual-discipline tracks.
🇸🇬 AI Singapore x NTU/NUS: Joint academic–government programs to embed AI in engineering and humanities alike.
🇺🇸 NSF AI Institutes: Embed educational components (K–PhD) alongside research mandates.
A nation cannot become AI-literate by elite expertise alone. Workforce AI training ensures that every economic sector—from logistics and education to construction and customer service—has the ability to interface with, supervise, and collaborate with AI systems.
It’s not about turning everyone into an ML engineer; it’s about universal AI fluency. This is the horizontal expansion of AI competence, necessary for diffusion, absorption, and trust.
National AI Literacy Campaigns
Government-led programs that offer free or subsidized AI courses for all ages and industries.
Stacked Microcredentials & Modular Learning Pathways
Non-linear educational routes suited for upskilling and reskilling across sectors.
Sector-Specific AI Training
Tailored programs for teachers, healthcare workers, civil servants, factory managers, etc.
Public–Private Training Partnerships
Industry-led certifications aligned with national standards.
Integration into Vocational and Technical Schools
Workforce colleges and apprenticeships that focus on applied AI competence.
Mobile-first, language-localized AI courses and assessments.
Credential stacking: AI for HR, AI for operations, AI for public administration.
AI career path builders with job market alignment.
Inclusion-focused design for underserved or displaced workers.
🇸🇬 AI for Everyone / AI4Industry / AI4Kids: Government-funded universal training programs.
🇩🇪 AI Campus: Federated, open online AI training for public-sector workers, SMEs, and unemployed citizens.
🇯🇵 METI AI Human Resource Development Framework: Tracks skill levels across population segments.
🇺🇸 Good Jobs Challenge + NSF Workforce Hub Grants: AI upskilling tied to local industry needs.
AI systems must be proven in context before they are trusted at scale. Testbeds are controlled environments—real or simulated—where high-stakes AI deployments can be evaluated safely, audited rigorously, and calibrated for societal alignment.
This is the sandbox-to-society bridge: without it, deployment either stalls due to risk aversion or proceeds recklessly without safeguards.
Multi-Stakeholder Governance Models
Clear roles for regulators, developers, auditors, users, and affected communities.
Sectoral Focus Areas
Health, mobility, finance, education, criminal justice, and public administration.
Legal and Ethical Guardrails
Testing with opt-in consent, fallback mechanisms, transparency obligations.
Evaluation Metrics Beyond Accuracy
Fairness, robustness, privacy, explainability, human-in-the-loop efficacy.
Interoperability and Reusability
Open APIs, benchmark datasets, and modular testing protocols.
Urban test zones (smart cities, transport).
Synthetic populations for demographic robustness testing.
Open registries of tested systems with performance and risk reports.
Real-world simulations (e.g., policy modeling, disaster response AI).
🇪🇺 TEFs (Testing and Experimentation Facilities): Sector-specific EU-wide testbeds for healthcare, agri-tech, and manufacturing.
🇬🇧 CDEI’s Regulatory Sandboxes: Risk-mapped deployment environments for policing, finance, and online platforms.
🇸🇬 AI Verify: A globally available self-assessment toolkit for explainability, fairness, and robustness.
🇰🇷 Korea AI Verification Center: Certifies AI systems before market release based on standardized risk metrics.
Every nation has strategic sectors—be they agriculture, transportation, energy, water, or logistics—whose optimization yields macro-level gains. AI allows these traditionally slow, analog domains to become adaptive, predictive, and resilient. This is not generic automation—it is mission-tuned orchestration.
High-quality domain data (yield, grid loads, freight paths, school attendance)
Vertical AI partnerships (startups, research labs, ministries)
Digital twins or simulation environments for sectoral dynamics
Cross-agency data integration and interoperability mandates
AI procurement and regulatory fast-tracks for core infrastructure
Smart farming platforms (precision irrigation, pest prediction)
AI-augmented traffic systems (adaptive signaling, congestion forecasting)
Energy demand forecasting + carbon optimization tools
Regional digital service design based on predictive AI models
🇳🇱 Wageningen AI for Agri: Predictive soil and crop intelligence
🇸🇬 AI for Mobility: Integrated public transport load balancing and routing
🇮🇳 Kisan AI Platforms: Weather-informed agri advisories + market insights
🇰🇷 AI Power Grid Optimization: Real-time load-balancing with renewables integration
Health systems are data-rich, labor-constrained, and consequence-heavy. AI augments them not just by increasing throughput, but by redefining diagnostics, surveillance, and equity. It also anchors biosecurity, where AI can forecast outbreaks, simulate countermeasures, and triage crises.
Health data interoperability standards (EHR integration, privacy-preserving analytics)
Regulatory clarity for medical AI devices and decision-support systems
AI-trained clinical and public health professionals
Simulation and modeling platforms for outbreak forecasting, health equity modeling
AI for diagnostics: radiology, pathology, genomics
Bio-surveillance agents detecting early outbreak signals
Drug discovery copilots for molecule ranking and trial simulation
Resource optimization for hospital logistics and emergency response
🇺🇸 ARPA-H pandemic modeling platforms and GPT-powered R&D copilots
🇫🇷 Owkin: AI pathology + oncology prediction
🇸🇬 GovTech COVID AI: Real-time case forecasting and hospital load balancing
🇨🇳 P4 Digital Platforms: Real-time contact tracing and health code assignment
Courts, legal systems, and regulatory agencies are text-heavy, slow-moving, and resource-unequal. AI can deliver efficiency, access, and fairness—not by replacing judgment, but by accelerating comprehension, precedent navigation, and public access.
Digitized legal corpora: Court records, laws, regulations, filings
AI-augmented legal interfaces for citizens and paralegals
Human-in-the-loop review and audit mandates
Bias mitigation pipelines with systemic correction capability
AI tools for summarizing case law and legal documents
Virtual legal assistants for public-facing advice
Predictive analytics for judicial resource allocation
AI-supported sentencing or bail guidance under reviewable conditions
🇺🇸 DoNotPay: Legal GPT for consumer and traffic law
🇧🇷 Victor Project: Brazilian Supreme Court’s AI for case triage and similarity detection
🇪🇪 Veriff: Identity verification + e-residency via AI-compliant workflows
🇫🇷 Ministry of Justice pilots: Court assistant copilots for administrative cases
In moments of chaos—earthquakes, floods, pandemics, cyberattacks—state capacity is measured not in slogans but in latency, coordination, and foresight. AI gives nations fast perception, real-time inference, and cognitive augmentation under stress.
Geospatial, social, and environmental sensor integration
Emergency logistics platforms with AI routing and prioritization
Real-time communication copilots for first responders and hotline agents
Simulation systems for scenario planning and contingency modeling
Damage estimation from aerial/drone footage
AI-assisted hotline triage and multi-language response
Supply chain AI for distributing food, medicine, equipment
Early warning systems based on pattern detection
🇯🇵 Earthquake AI + Drone Imaging for structural collapse modeling
🇮🇳 SEEDS AI Platform for community-level climate resilience planning
🇺🇸 FEMA AI prototypes for disaster logistics + misinformation mitigation
🇸🇬 Smart Nation emergency dashboards for pandemic/crisis visualization
Education is the root system of national competence. AI allows mass education systems to become adaptive, context-sensitive, and equity-forward. It replaces one-size-fits-all curricula with responsive, modular, learner-centered trajectories.
Digital infrastructure in schools (devices, connectivity, dashboards)
Curriculum-as-data frameworks for semantic analysis and adaptation
Teacher AI tools for personalized guidance, grading, and scaffolding
Governance rules for bias, safety, and explainability in learner models
National tutoring copilots for math, language, history
Adaptive learning platforms that personalize sequence and pace
Dropout prediction + student support triage systems
Generative tools for creative learning and interdisciplinary exploration
🇺🇸 Khanmigo: GPT-4-based tutor rolled out in U.S. school districts
🇸🇬 AI for Learning Platform: Centralized, student-specific trajectory designer
🇺🇳 UNICEF Learning Passport AI pilots: Personalization for displaced learners
🇧🇷 CAEd AI tools: Adaptive learning analytics for public school performance
AI isn’t just for startups and science—it's a governing infrastructure. Governments can use AI to augment policymaking, automate bureaucratic functions, forecast economic and social outcomes, and provide more personalized, responsive, and intelligent public services.
This is where nations "eat their own AI cooking"—proving that public sector adoption isn’t just viable, but transformational.
AI-literate civil service with procurement and deployment capacity
Ethical and operational governance frameworks for AI in state systems
Central digital services unit (GovTech, digital ministry, etc.)
Open data infrastructure to feed government AI
Civic engagement mechanisms for feedback and accountability
AI copilots for tax filings, benefits applications, licensing, and inquiries
Public sector RAG systems to answer legal, procedural, or historical queries
AI systems for dynamic budgeting, infrastructure forecasting, policy modeling
Automated document processing, translation, and classification at scale
🇸🇬 GovTech Singapore: Chatbots, AI document processors, digital assistants in every agency
🇺🇸 IRS + AI: NLP-based support and fraud detection
🇪🇪 e-Estonia: Fully integrated, AI-assisted e-governance from birth to death
🇧🇷 Public Procurement AI: Risk detection for fraud in supplier ecosystems
Most nations are rich in domain-specific scientific talent but lack the tools to scale research velocity, synthesis, and discovery. AI can act as a cognitive multiplier across all disciplines—supercharging hypothesis generation, experimental design, and knowledge integration.
This is not just about making researchers faster. It’s about opening new epistemic terrain.
Access to foundational scientific LLMs and multimodal AI (text + code + structure + image)
Digitized national research libraries and data lakes
Science-focused compute credits and AI tooling grants
Cross-disciplinary teams of domain experts + ML engineers
New research workflows integrating AI into literature, modeling, lab automation
AI copilots that generate, refine, or refute hypotheses
Autonomous agents to run literature reviews, simulation sweeps, parameter optimization
LLMs that write, translate, and refactor academic papers
AI-assisted lab notebooks and scientific process planning
🇺🇸 ARPA-H + GPT-4 for biomedical synthesis and experimental design
🇬🇧 DeepMind’s AlphaFold: Protein folding prediction accelerating life science R&D
🇫🇷 Sanofi x Exscientia: AI-driven molecule discovery and repurposing
🇨🇦 Amii + Vector + MILA: Embedding AI into climate modeling, neuroscience, and epidemiology
AI can no longer be siloed to national productivity. Climate change, pandemics, poverty, misinformation, and biodiversity loss are planetary-scale problems—and AI is one of the few technologies with the complexity-matching capacity to address them.
This layer moves AI into the realm of civilizational coordination.
SDG-aligned funding models and research missions
Cross-national collaboration platforms and open data exchange
Global compute and model-sharing protocols
AI governance structures that empower Global South participation
Regulatory harmonization for cross-border deployment
Climate forecasting AI for emissions, droughts, floods
Epidemic early warning and outbreak containment modeling
Food insecurity and supply chain resilience systems
Misinformation detection at platform and geopolitical scale
🇺🇸 AI for Earth (Microsoft): AI tools for climate data and conservation
🇪🇺 AI for Green Deal: Funding AI for emissions modeling, urban heat mapping, disaster response
🇸🇬 AI for Smart Agriculture: Regional collaboration across ASEAN
🌍 UN Global Pulse AI Labs: AI applied to migration, poverty, and crisis prediction