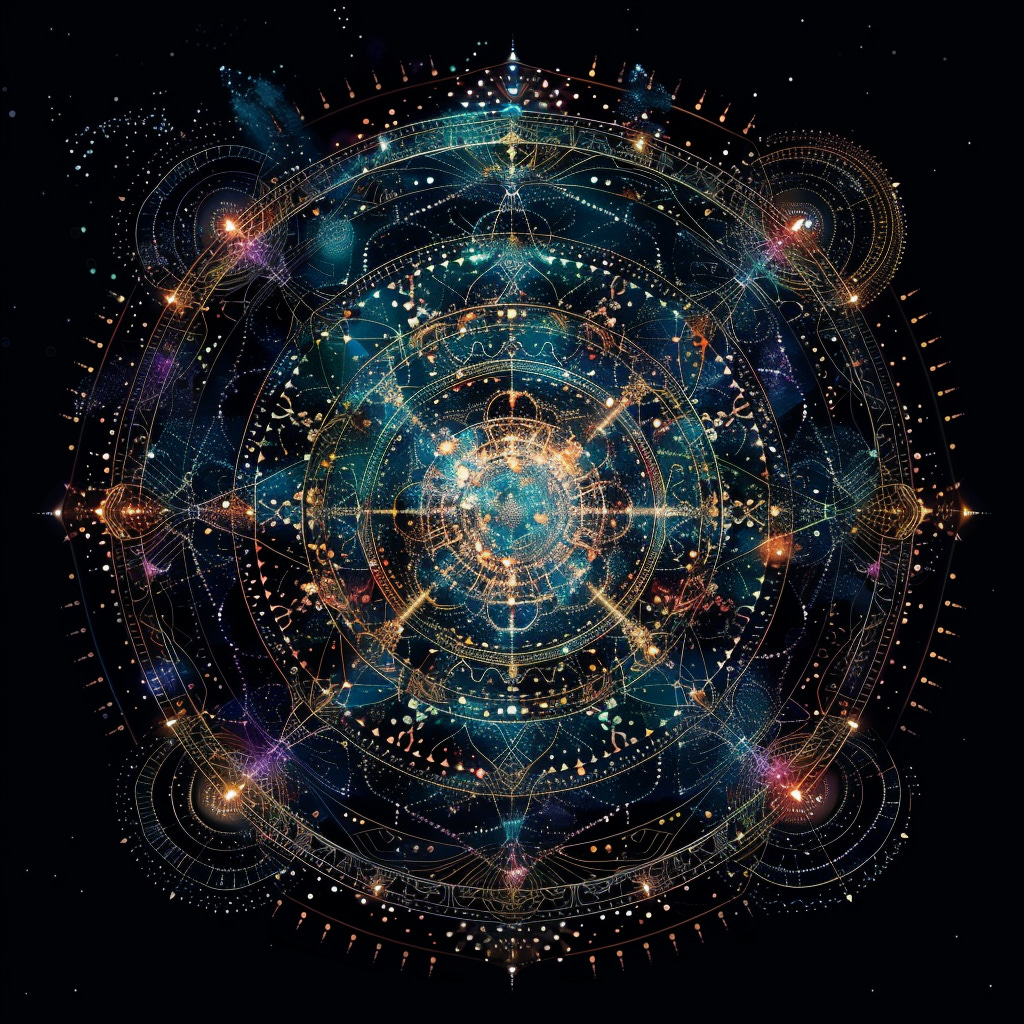
April 11, 2025
In an age where intelligence is no longer the sole province of humans, the Chief AI Officer (CAIO) emerges not as a technical role, but as a profound evolution of executive function itself. The CAIO is not simply a manager of systems—but a governor of cognition, an architect of symbiosis between machine reasoning and human enterprise. As AI becomes foundational to decision-making, product design, culture, and even ethics, the CAIO becomes the one entrusted with curating, commanding, and continuously reshaping how intelligence lives within an organization.
Yet this role is unlike any before it. The CAIO inherits the disciplines of classical leadership—strategic thinking, team-building, decision-making—but must also radically transform them. Traditional managerial tools become inadequate when decisions are probabilistic, when feedback is algorithmic, and when the "team" includes synthetic agents with neural cores instead of egos. What once were operational skills must now evolve into cognitive strategies. The question is not merely “What should a CAIO know?” but “How should a CAIO think, design, and evolve?”
This article explores twenty foundational skills—transformed, reframed, and reimagined for the age of AI. Each is rooted in traditional executive wisdom but now contorted through the lens of recursive systems, multi-agent environments, and rapidly shifting ontological ground. These are not just job requirements. They are disciplines of mind and architecture. They represent how the CAIO navigates complexity, distributes thinking, engineers alignment, and instills meaning into systems that increasingly learn and act on their own.
More than technocratic fluency, the ideal CAIO requires strategic sentience: the ability to see where intelligence flows, where it stagnates, where it misaligns, and where it accelerates value. They must speak both in the abstract language of cognition and the grounded logic of business transformation. They are philosopher, engineer, diplomat, and provocateur. To wield this role well is to reimagine the enterprise—not as a collection of departments, but as a network of evolving intelligences.
What follows is a synthesis—a scaffold of twenty critical capabilities that every aspiring CAIO must develop and transcend. These are not merely tips or frameworks; they are design imperatives for operating at the frontier of enterprise intelligence. This is not the future of management. This is its reinvention.
Traditionally, managers set goals to give clarity, direction, and purpose. For you, the CAIO, goals are not endpoints—they are evolving intent vectors, constantly recalibrated by models, data shifts, and emergent possibilities. You must shape living goals that can think back.
In old paradigms, this meant picking the highest-leverage task. In your world, it's not task triage—it’s constraint orchestration. You manage latency, cost, ethics, context windows, and attention bandwidth simultaneously. You are the conductor of intelligent tradeoffs.
For traditional managers, hiring was about filling roles. For you, it's about composing an ecosystem of minds—some biological, some artificial. You decide which parts of cognition are human, which are synthetic, and which are hybridized.
Historically, feedback helped shape people’s growth. But you must also give feedback to systems, models, pipelines. You manage a recursive network of feedback loops—where performance is observed, learned from, and encoded into both culture and code.
Delegation once meant trust. For you, it means cognitive allocation. What gets automated, what gets prompted, what remains a human judgment? You don’t just assign tasks—you distribute intelligence across a cognitive stack.
Meetings were places to align and inform. But now they are synchronization rituals between humans and machines. Dashboards speak. Agents summarize. Insight competes with overload. You must choreograph signal through multiple substrates.
For the manager, it was about appraisals. For you, it’s about measuring augmented capability—how well teams operate in tandem with AI. You're managing human performance in AI-enhanced contexts, tracking uplift, friction, and interface breakdowns.
In the past, conflict was interpersonal. Now, it’s inter-ontological. A human says "this is unethical"; the model says “it's optimal.” Your role is to engineer alignment—between human values, machine logic, and enterprise goals.
A manager weighs options. You simulate futures. You model uncertainty, run predictions, generate counterfactuals, and interpret probabilities. Your decisions are multi-agent consensus structures, not just instinct with spreadsheets.
In the past, communication meant clarity. Now, it’s translation across ontologies. You speak “boardroom”, “engineer”, “regulator”, and “model prompt”. You harmonize semiotic layers into one coherent flow of action.
Planning was about forecasting. But the CAIO doesn’t just forecast—they create recursive strategy engines that learn. You build plans that can observe themselves, adapt, and reconfigure as models evolve.
Previously, trust was a human affair. Now, it includes machine behavior. You must foster belief not only in you—but in the intelligence systems under your command. You are responsible for ethical transparency, explainability, and epistemic humility.
A breach, a blackout—these still happen. But in your world, failure is often silent, synthetic, probabilistic. You must design containment architecture—so when models misbehave, hallucinate, or bias creeps in, the damage is localized, legible, and reversible.
Culture used to be “how we do things here.” You now craft hybrid cultural scaffolding—where humans and AIs work, think, and learn together. You shape the protocols of interaction, the language of collaboration, and the rituals of shared cognition.
Classic coaching focused on human growth. Now, you coach both humans and models. You coach teams on how to co-think with algorithms—and you coach models to behave, align, and support. Your style is recursive. Your goal is symbiotic mastery.
Traditionally this meant budget and headcount. You must now allocate cognition—compute power, prompt tokens, model bandwidth, attention. You’re balancing neural cost curves against strategic returns. Your currency is insight-per-second.
Old-school problem-solving meant root cause analysis. You now trace systemic causality across entangled agents. Errors may come from data drift, misalignment, prompt ambiguity, or human misuse. You debug multi-agent cognition itself.
Inspiration was speech and sentiment. Now, it's alignment across moral, strategic, and aesthetic dimensions. You must connect the Why of intelligence to both code and conscience. You must help teams feel the future they're shaping.
Before, this was about process and headcount. Now it’s scaling cognition. You build AI-native architectures, model pipelines, and reusable patterns of synthetic thought. You scale by building platforms of learning.
Patience was once restraint. Now it is temporal risk engineering. You don’t just delay—you create optionality. You bet on multiple futures, design experiments, and hedge across paradigm shifts. You wait with structured readiness.
Goal setting has always been about establishing clear, measurable objectives that guide action and alignment. Peter Drucker’s ghost still looms with his classic SMART goals, and Grove championed OKRs—Objectives and Key Results—as a way to manufacture clarity and track performance velocity.
The CAIO doesn’t merely set goals—they design intent structures that co-evolve with algorithmic systems. Why? Because AI introduces non-linearity, feedback loops, and recursive improvement. A static goal is obsolete the moment a model starts learning.
Intent Vectors: High-dimensional goals that guide AI-human ecosystems, not just people.
Performance Guardrails: Outcomes are measured via model accuracy, ethical boundaries, and behavior in edge cases.
Dynamic Adjustment Protocols: Systems learn, environments shift. Goals must reconfigure themselves mid-flight.
Prompt Objectives: Training LLMs, not just teams, to “understand” strategic direction through instruction design.
Andrew Grove built management around measurable production. For the CAIO, that becomes measurable intelligence output.
Eric Ries insists that goals should trigger validated learning, not just vanity metrics. This pairs perfectly with model iteration loops.
Ray Dalio would frame this as systematizing your intent to be algorithmically replicable. If your strategic aim can’t be expressed in logic, it’s fantasy.
Classic prioritization is about choosing what to do first, based on urgency, value, and capacity. Eisenhower matrices, Kanban boards, 80/20 rules. The language is always: “Focus. Cut. Sequence.”
In the AI ecosystem, constraints—not choices—become your control surfaces. You are no longer asking “What should we do?” but “What can we afford to compute?” “What can we explain?” “What regulatory fire can we survive?”
Computational Budgeting: Choosing what models to run given cost and speed tradeoffs.
Ethical Constraint Mapping: Not just “can we” but should we—and how do we audit that decision?
Latency vs. Accuracy Tradeoffs: Prioritizing across competing AI performance variables.
Temporal Sequencing: When to release, iterate, or delay based on model maturity.
Claire Hughes Johnson prioritizes by task triage and organizational phase. For a CAIO, this becomes systems triage: what intelligence is deployed now, versus sandboxed for later.
Grove emphasized limiting managerial bandwidth to high-leverage actions. The CAIO does this at scale with AI-first opportunity cost analysis.
Jim Collins would demand alignment with the Hedgehog Concept: doing only what intersects with your unique advantage and economic engine.
Hiring used to mean: find a competent, culture-fit human for a role. Lencioni’s team dysfunctions made clear the cost of hiring for skill and ignoring team chemistry. Grove pushed hard for task-relevant maturity.
Now you’re not just hiring people—you’re building a distributed cognition mesh. You must decide:
What humans should do.
What AI should do.
What humans and AIs should co-do.
Human + Synthetic Division of Labor: You hire capabilities, not just CVs.
Promptcraft + Model Whispering: Human hires must be able to shape AI outputs, not just perform solo.
Adaptive Role Structures: Roles morph based on model capabilities. HR becomes cognitive topology design.
Synthetic Agents as “Team Members”: LLMs writing code, answering tickets, doing research. These are entities to be managed, not tools.
Dalio: “The WHO is more important than the WHAT.” In CAIO terms, the WHO may now be GPT-5.
Claire Hughes Johnson redefines onboarding as system calibration. New hires learn how to collaborate with algorithms, not just peers.
Ries would demand that every hire be tied to a learning hypothesis: “How does this hire accelerate the machine?”
Traditionally, feedback is a mirror: periodic, qualitative, subjective. The best managers give hard truths early and often, and build a culture of candor and growth. Dalio made radical transparency the gospel. Grove saw feedback as an instrument for output maximization.
In an AI-powered world, feedback becomes a signal calibration loop across humans, models, metrics, and behavior. It’s not about what someone did last quarter. It’s about what the system is doing right now, and how we tune it—ethically, behaviorally, and cognitively.
Synthetic Feedback Loops: AIs feeding performance signals to users, leaders, and each other.
Cross-Signal Interpretation: Emotional sentiment, model confidence scores, NPS, hallucination rates—all signals must be converged and contextualized.
Self-Feedback Infrastructure: Humans using AI to reflect on their own behavior. Think: an LLM coach embedded into your leadership rituals.
Organization-as-Feedback-System: Every behavior logged, every insight timestamped, every mistake modeled for future immunity.
Dalio built Bridgewater’s success on real-time, radical feedback loops between beliefs and behaviors. The CAIO operationalizes that across humans and algorithms.
Lencioni argues that feedback must flow before results drop. For the CAIO, the system must detect weak signals before anyone even notices.
Claire Hughes Johnson reminds us: Feedback is a design input, not just a performance post-mortem.
Delegation used to be about entrusting a person with responsibility. Grove spoke of maximizing managerial leverage—handing tasks downward to boost throughput. Trust, clarity, and accountability were the currencies.
The CAIO does not delegate tasks—they allocate cognition across humans and machines. Delegation becomes a dynamic orchestration of who or what should think, decide, act, or refine.
Task Disaggregation: Break tasks into micro-intents that can be partially fulfilled by AIs.
Agent Assignment Protocols: Decide when to hand off work to LLMs, when to embed copilots, and when to leave it human.
Feedback-Reinforced Delegation: Systems learn from prior success/failure of delegation decisions.
Accountability Mesh: Responsibility is tracked across machine + human agents with transparency.
Dalio: “Design a machine.” Delegation for the CAIO is mechanism design, not people pleasing.
Claire Hughes Johnson: Delegation requires role clarity and trust—but now that includes AI roles too.
Patrick Lencioni: Without clarity, delegation breeds blame. The CAIO must define interface contracts between agents.
Meetings were where alignment happened. Grove dissected them by type: decision meetings, one-on-ones, process updates. Leaders used them to disseminate clarity and absorb signal.
The CAIO presides over meetings that include machines—dashboards that speak, models that simulate, GPT agents that summarize and challenge decisions. Meetings are no longer calendar events—they’re cognitive synchronization rituals.
Pre-synced Context Windows: AIs ingest context and generate insights before meetings start.
Dynamic Meeting Agents: Tools like LLMs offer in-meeting recommendations, live analytics, error checking.
Output as Artifact: Every meeting yields a structured, tokenized, queryable summary for humans and machines.
Human-Machine Role Assignment: Who runs the decision? Who interprets the model?
Grove: A meeting is a managerial “production line.” The CAIO runs it like an intelligence factory.
Eric Ries: Meetings must trigger learning, not just reporting. The CAIO uses each session as an experiment.
Claire Hughes Johnson: Document everything. A CAIO does it modally: text, voice, summary, prompt snippet.
Classic performance management was review-centric. You assessed output, gave feedback, adjusted roles. Grove demanded objectivity. Dalio demanded brutal honesty. Collins sought results over charisma.
You are no longer evaluating only people. You’re evaluating how intelligence is distributed, amplified, or degraded across systems. Human performance must be judged in tandem with AI augmentation.
Human-Machine Pair Evaluation: Is the team better with the model, or despite it?
Cognitive Load Assessment: Who is being overwhelmed or under-leveraged by the AI stack?
System-Wide Output Tracing: From raw input to decision, which part of the intelligence chain faltered?
Longitudinal Learning Curves: Are humans becoming smarter through AI interaction?
Dalio: “Don’t confuse output with potential.” For the CAIO, raw output is insufficient. You need elevated cognition.
Claire Hughes Johnson: Differentiate by slope, not just speed. Who’s adapting to the AI era, not just surviving it?.
Grove: Performance is leverage. CAIOs now measure leverage across biological and artificial actors.
In traditional orgs, conflict navigation was emotional jujitsu: surfacing disagreements, preventing passive-aggression, resolving team rifts. Lencioni made trust and vulnerability the preconditions to healthy conflict.
CAIOs face a new geometry of misalignment:
Humans vs. AIs
Different AI models competing in optimization
Regulatory vs. business incentives
AI hallucination vs. human assumption
You are not resolving interpersonal drama—you are engineering semantic harmony across layers of meaning and models.
AI-Human Conflict Detection: Is the system contradicting human ethical boundaries or operational beliefs?
Model-to-Model Alignment: Does your forecasting engine agree with your recommender system?
Governance-Aware Decisions: Conflict is often rooted in hidden risk exposure. Make it legible.
Socio-technical Mediation: Facilitate dialogue between groups of humans with different AI dependencies.
Lencioni: Conflict thrives when trust dies. The CAIO builds machine trust as well—through explainability and version transparency.
Simon Sinek: People align when the why is clear. The CAIO must articulate purpose to both people and prompts.
Dalio: Conflicts are opportunities to understand. CAIOs build systems that not only resolve tension—but learn from it.
Managers make decisions by gathering data, consulting stakeholders, and choosing an action with confidence and timeliness. Dalio called for believability-weighted decision-making, and Grove emphasized decisions that maximized throughput.
The CAIO does not just decide—they simulate futures, using AI models to predict outcomes, assess second-order effects, and model unintended consequences. Decision-making becomes pre-decision computation.
Agent-Based Simulation: Running AI-driven projections of how customers, systems, or regulators will react.
Causal Inference: Understanding not just correlation but why something causes something else.
Uncertainty Mapping: Every decision includes quantified unknowns, risks, and model limitations.
Collaborative Decision Loops: Include machine agents in the deliberation—AI generates perspectives, not just numbers.
Dalio: The best decisions are algorithmizable. The CAIO writes logic trees into reality.
Eric Ries: Decisions are experiments. The CAIO makes every big choice falsifiable.
Collins: Great companies have a “Stop Doing” list. CAIOs need a “Don’t Predict, Simulate” list.
Communication was clarity, persuasion, and alignment. Sinek: Start with Why. Claire Hughes Johnson: “Say the thing you think you cannot say.” Managers were trained to cascade clarity and direction down the org stack.
Communication becomes multi-ontology harmonization: the CAIO must speak human, speak data, speak machine. This is less about “telling” and more about synchronizing intelligences.
Narrative Layering: Explaining decisions in formats digestible by execs, engineers, regulators, and models (prompt design is storytelling).
Insight Translation: Turning complex model outputs into causal insight without hallucination or oversimplification.
AI-augmented Messaging: Use models to synthesize, test, and tailor messaging to different internal agents.
Trust-Centric Cadence: Frequency and transparency of communication as a design artifact.
Sinek: CAIOs must wield the “Why” to bind machine action to human purpose.
Claire: Managers turn implicit into explicit. CAIOs turn opaque model cognition into shared understanding.
Lencioni: Great teams overcommunicate trust. The CAIO makes trust machine-readable.
Planning is where time becomes strategy. It’s OKRs, roadmaps, and Gantt charts. Grove treated it like resource allocation across timelines. Managers used plans to lock intent into structure.
The CAIO cannot rely on fixed plans. Why? Because the intelligence environment evolves recursively. Models learn, data shifts, capabilities reconfigure. Planning becomes a self-updating system—a living logic graph.
Recursive Planning Engines: Strategies are code that refactor themselves when new data or conditions appear.
Contingency as Norm: “If-then” logic isn’t optional—it’s your skeleton.
Outcome Horizons: Short-term exploitation vs. long-term exploration—each with distinct AI augmentation.
Data-Driven Planning Drift Detection: Plans self-monitor their relevance.
Grove: Today’s actions are tomorrow’s outputs. The CAIO sees outputs as evolving organisms.
Claire Hughes Johnson: Planning sets “operating cadence”—for the CAIO, cadence is code.
Ries: Plans should be minimum viable strategy until proven. The CAIO A/B tests the roadmap.
Trust used to mean follow-through, transparency, vulnerability. Lencioni made it the base of every functional team. Without it, everything else—commitment, accountability—collapsed.
Trust must now scale across humans, models, and systems. People don’t just need to trust you—they need to trust black box models, predictive analytics, LLM decisions. Trust becomes engineered into cognition.
AI Explainability: Can users understand what the model is doing—and why?
Transparency Rituals: Regular model behavior audits, ethical disclosures, failure post-mortems.
Vulnerability Modeling: The CAIO shares uncertainty, not just direction—“Here’s what we don’t know yet.”
Cognitive Safety Design: Build systems where humans can override, challenge, or re-steer AI confidently.
Lencioni: Without trust, everything decays. CAIOs build trust at the model-interface layer, not just interpersonal.
Dalio: Radical transparency isn’t just honesty—it’s systemic clarity. Trust is knowing how the machine thinks.
Simon Sinek: If the “why” is hidden, suspicion grows. The CAIO makes why-decisions visible to humans and machines alike.
Managers were trained to respond to crises with clarity, decisiveness, and calm. Grove described how Intel exited DRAM under extreme pressure—a masterclass in decisive adaptive focus. The skill was to keep the org moving while bleeding.
In the world of AI, crisis isn’t just reputational or financial—it’s cognitive failure, model drift, or ethical rupture. The CAIO must anticipate and architect containment: fail-safes for synthetic errors and recovery pathways for cascading misalignments.
Failure Mode Prediction: Scenario-modeling for hallucinations, adversarial prompts, data poisoning, and drift.
Kill-Switch Design: Systems must be interruptible by humans—both technically and organizationally.
Forensic Intelligence Tooling: Real-time post-mortems on where and how the failure occurred.
Cross-System Containment Protocols: Isolating errors in one subsystem from infecting others.
Grove: When the storm comes, fall back to your core strength. The CAIO defines that strength in terms of synthetic reliability.
Ries: Crisis reveals what should’ve been tested earlier. The CAIO builds resilience via MVPs.
Dalio: The worst failure is one that doesn’t teach you. The CAIO builds self-teaching crisis memory into the system.
Culture was the shared air people breathed—values, rituals, language. Sinek made purpose central. Lencioni insisted trust, commitment, and clarity weren’t optional; they were culture.
The CAIO is responsible for designing a culture where humans and machines co-shape outcomes. Culture is not just social—it’s semiotic and cognitive. The goal isn’t "company values." It’s alignment across divergent reasoning substrates.
Cognitive Norms: How humans and AIs collaborate—when to defer, when to override, when to debate.
Shared Interpretive Frames: Creating common ground across departments, APIs, and LLMs.
Narrative Infrastructure: Purpose, ethics, and decision rituals encoded into prompts, dashboards, team behaviors.
Symbolic Alignment: Training models and humans to respond to the same triggers with shared meaning.
Sinek: The CAIO’s “Why” must resonate with humans and be embedded in model training paradigms.
Claire Hughes Johnson: Culture is enforced through cadence and clarity. The CAIO sets cultural API boundaries.
Dalio: Culture is protocol. The CAIO implements values as executable logic.
Managers were coaches: unlocking potential, delivering hard feedback, nurturing trajectory. Grove believed coaching multiplied output. Claire Hughes Johnson stressed individualized development feedback.
Now, coaching is not just for people. The CAIO is coaching humans and AIs—helping teams learn how to learn with models, and helping models learn to align with humans. Coaching becomes recursive learning loop design.
Human Capability Shaping: Training humans to co-think with AI—prompt literacy, model trust calibration, judgment sharpening.
AI Model Tutoring: Reinforcement tuning, feedback loops, and behavior shaping via human-in-the-loop systems.
Learning Velocity Optimization: Maximize how fast the org gets smarter per unit of experience.
Meta-Coaching: Coaching humans on how to coach with AI at their side.
Grove: The best managers grow people. The CAIO grows organisms of augmented cognition.
Dalio: Coaching isn't hand-holding—it’s calibration. The CAIO builds systems that self-calibrate.
Ries: Learning is the only KPI that compounds. The CAIO installs feedback as architecture.
Resource allocation is the deployment of time, money, and people toward the highest-return activities. Grove and Collins stressed ROI thinking and brutal prioritization.
Time and money still matter—but now so does compute, data liquidity, and human attention span. The CAIO must allocate across cognitive bottlenecks. You budget not just dollars—but thinking.
Compute Allocation Strategy: GPUs and token budgets are finite. What gets model time? What doesn’t?
Context Window Prioritization: What fits in the prompt matters. Compress the trivial, expand the strategic.
Human Attention Budgeting: Teams are distracted by alerts, dashboards, models. The CAIO throttles noise-to-signal.
Latency vs. Cost Tradeoffs: Do we run in real-time or batch? Optimization now involves epistemic cost curves.
Grove: Management is leverage. The CAIO’s leverage is computational cognition.
Dalio: Every resource you allocate reflects your principles. The CAIO must define what deserves intelligence.
Collins: Discipline is saying “no” to 1,000 good ideas. The CAIO applies this to API calls, not just projects.
Managers were taught to dig past symptoms to uncover root causes. Grove insisted on logic trees. Dalio had a 5-step loop for diagnosing and solving problems with rigorous reflection.
For the CAIO, diagnosis transcends organizational dysfunction—it becomes the art of tracing invisible, often statistical, causality across hybrid systems. You’re debugging the behavior of humans, algorithms, interfaces, and datasets all at once.
Model Behavior Analysis: Identify whether failure emerged from misalignment, poor training data, hallucination, or deployment context.
Human-AI Interaction Pathways: Did the issue arise from misunderstanding the AI—or the AI misreading its instruction?
Data Drift Monitoring: Ensure that slow, silent decay of model performance doesn’t become a systemic blind spot.
Causal Graphing: Map decision failures across time, agents, and abstraction layers.
Grove: Don’t solve the smoke, find the fire. CAIOs must ask: “Where did the cognition collapse?”
Dalio: Pain + reflection = progress. The CAIO builds diagnostic reflexes into the system.
Ries: Ask “Why?” five times—but also across five different models. Each sees a different layer.
Great leaders inspired. Sinek taught us to start with why. Inspiration turned strategy into meaning, and meaning into movement.
The CAIO must inspire in a world where the workforce is hybrid, where machines must be directed, and humans must be uplifted. Inspiration becomes not just motivational—it is alignment between purpose, cognition, and the aesthetics of decision-making.
Narrative Precision: Ensure your story of “why AI” resonates across fears and functions.
Ethical Fluency: Inspire trust by standing for a principled intelligence future.
Symbolic Framing: Use language, interfaces, and even visual design to embody coherence.
Purpose Embedding: Inject strategic intent into models, culture, and product alike.
Sinek: People don’t buy what you do—they buy why you do it. The CAIO makes sure models do too.
Lencioni: Without inspiration, trust dries up. The CAIO maintains emotional resonance even through dashboards.
Claire Hughes Johnson: Inspire with structure. Even rituals can carry meaning.
Scaling was about adding people, process, and infrastructure. Claire Hughes Johnson obsessively detailed scaling culture through clarity, while Ries emphasized scaling learning loops.
For the CAIO, scaling means designing platforms that multiply cognitive leverage. You’re scaling not just products or people—you’re scaling organizational intelligence.
Reusable AI Infrastructure: Create base models and pipelines that can serve multiple business lines.
Toolchain Abstractions: Build layers that allow teams to plug into AI without reinventing interfaces.
AI-Native Operating Models: Your org must operate at the speed of data.
Org-wide Prompting Patterns: Develop shared language structures so employees can think with AI coherently.
Claire Hughes Johnson: Scaling is clarity multiplied by repetition. CAIOs do this through APIs and knowledge graphs.
Ries: Scalability is a side-effect of validated systems. CAIOs think in intelligence units, not departments.
Grove: Production scales through systems, not effort. For the CAIO, effort is cognitive—systems are synthetic.
Managers are told to “play the long game.” Collins’ “Flywheel” model is about cumulative momentum, not flashy sprints. Strategy required holding the line.
In the age of AI, strategic patience is not about waiting. It’s about temporal hedging—balancing today’s deployables with tomorrow’s breakthroughs. The CAIO builds optionality, not delay.
Dual Horizon Management: Run Horizon 1 (exploitation) while seeding Horizon 3 (moonshots).
Option Stack Design: Invest in capabilities that open future pathways—even if not profitable yet.
Innovation Sandboxes: Create low-risk zones to test bleeding-edge models.
Timing Reflexes: Knowing not just what to do—but when the world is ready for it.
Collins: Great companies push the flywheel. The CAIO knows where the AI flywheel is spinning—and where it’s still stuck.
Dalio: Don’t confuse activity with progress. CAIOs know when not to ship the shiny thing.
Ries: Be patient with vision, ruthless with validation. The CAIO lives inside this paradox.